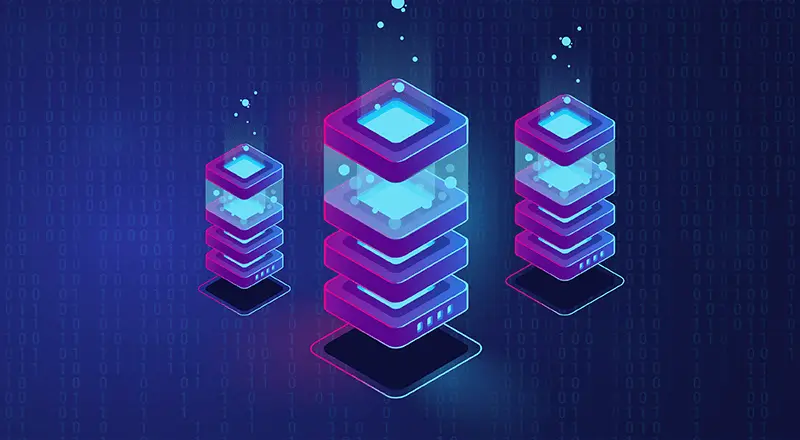
As enterprises adapt to new technologies to manage large scale, complex and fragmented business units, tons of data continue to be generated. This data comes from various sources such as customer interaction, social media, sales cycles, business transactions and so forth. Enterprises are therefore weighed down by these massive amounts of data that can run into petabytes and continues to grow at a rapid rate. The importance of this data cannot be undermined. and Gartner in its report, `Top Priorities for IT: Leadership Vision for 2021-Data and Analytics Leaders says, `Data literacy will become an explicit and necessary driver of business value, demonstrated by its formal inclusion in over 80% of data and analytics strategies and change management programs’.
Data Fragmentation Remains a Challenge for Business Enterprises
All this data is fragmented and can add significant value to business only when it can be processed cohesively, interactively and speedily to empower business decisions – decisions that can be applied to customer relationships, forecasts, and business transformation. However, even as enterprises gather more and more data, the challenge has been to collate fragmented and distributed data sources and bring them together.
Over the years, data warehouses have been helping businesses in decision making and intelligence. But typically, data warehouses work well with structured data. However, the challenge for enterprises is, their data is mostly unstructured, of high volume and variety. To overcome the limitations of data warehouses nearly a decade ago, a new concept of data lakes was created to store data, irrespective of format. But here again, data lakes come with their own limitations. They store data but are not well positioned in terms of imposing data quality or supporting data related transactions. As a result, once again, these vast volumes of data have become the personal nightmares of data scientists.
Even as enterprises to remain competitive in a digitally transforming world, look at their data volume and wonder how to make the best use of it, data scientists have more technical concerns. They need a platform that can apply SQL analytics, monitor the data, if possible, in real time and use this for machine learning. Sensing the need there have been companies that have launched highly intuitive and advanced AI solutions that look at unstructured content in conjunction with structured data sets. However, data warehouses that they already have may not be compatible with these AI solutions. Hence, enterprises to make the best of a bad situation, typically invest in multiple data storage and AI solutions including data warehouses and data lakes etc. But when it comes to unifying all these data sources and applications, there is a challenge and data scientists’ resort to the old method of copying data and pasting them, to move them from one system to another. But this process can be cumbersome, time consuming and expensive. More importantly, the required intelligence from these sources will not be available at the right time for decision makers rendering this data and its ensuing efforts almost useless.
Into the Future
A new next generation innovation is the lakehouse which takes the best features of data warehouses and data lakes to offer a standardized open-source platform that transforms data stored in data warehouses to form a structure which can therefore be used for machine learning.
Lakehouses support transactions, i.e. reading data and writing it at the same time. It supports schema to rationalize data, empowers BI and is open but standardized. More importantly it supports both structured and unstructured data which essentially means disparate data storehouses can be uniformly brought into this single platform. It supports various workloads and streams data in real-time.
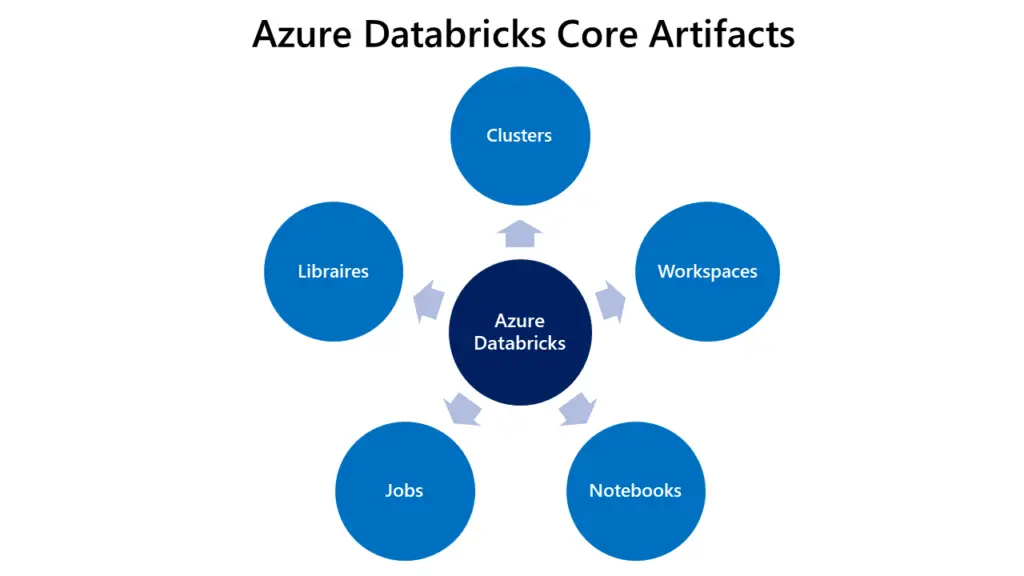
Integration and Uniformity – Key to Machine Learning
Once again lakehouses as a concept is interesting but enterprises require lakehouses that are integrated with their cloud service to ensure that there is overall manageability of data. For example, it is an undeniable fact that several enterprises that generate data use Microsoft Azure, cloud computing service for building, testing, deploying, and managing applications and services through Microsoft-managed data centers. According to statistics, 95% of Fortune 500 enterprises, of all sizes and maturities rely on Azure for cloud services in their digital transformation. For these enterprises it is critical to have a data processing platform that is integrated with Azure as it will help to consolidate all their applications and the resulting data in one platform.
What is Azure Databricks?
Solving the challenge of converting data into machine learning collaboratively, the new Azure Databricks platform, a result of a well thought out collaboration between Apache Spark from Databricks and Microsoft Azure has become a necessity for data scientists and analysts who wish to analyze data fast in an efficient, secure, and collaborative manner. This cloud-based platform simplifies data processing and empowers enterprises to derive the best benefits from their existing data. To simplify it is a single platform to unify all data, analytics, and AI workloads. Today’s data leaders must look at the entire data and machine learning landscape when considering new solutions.
Azure Databricks Architecture
Azure Databricks is structured to enable secure cross-functional team to collaborate while keeping a significant amount of backend services managed by Azure Databricks so you can stay focused on your data science, data analytics, and data engineering tasks. Although architectures varies depending on custom configurations (such as when you’ve deployed a Azure Databricks workspace to your own virtual network, also known as VNet injection), the following architecture diagram represents the most common structure and flow of data for Azure Databricks.
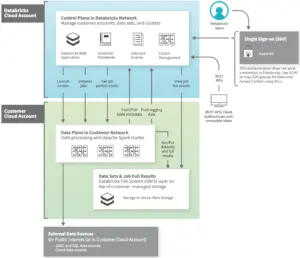
Source: Microsoft
Features of Azure Databricks
- Workspace – Databricks in true Microsoft fashion, offers a collaborative and interactive platform where data owners, stakeholders and business heads can work together. This feature of Databricks ensures that data is not a standalone by-product of businesses but the very nucleus of business transformation.
- Runtime – especially for huge workloads can be challenging. However, Apache Spark with constant updates and added components helps to ensure maximum performance while ensuring security.
- Integration – as all CISOs would agree is key to seamless performance and standalone platforms or applications, irrespective of their value, become cumbersome for IT heads. Azure Databricks integrates smoothly with Azure services, Apache Kafka and Hadoop storage. These integrations are purposeful to help enterprises to convert data into machine learning and Power BI, etc.
- Azure Databricks Security – Azure Databricks uses several tools to secure your data and network infrastructure. Learn more about the enterprise grade security in Azure Databricks.
Real-life example of Azure Databricks
To understand the business benefits of Databricks, let us visualize the case of a technology company in the media and entertainment segment. The company connects people to personalized experiences and as a result must have an astute understanding of customer needs and behavior. The company typically handles billions of individual interactions and at the same has to offer personalized experiences to every single one of them. The data runs into petabytes and with the help of Databricks this data was converted into data sets and optimized for learnings. With its collaborative ability stakeholders across teams could access the learnings and ensure that their goals of delivering personalized interactions with customers could be easily attained.
Azure Databricks Pricing
Azure Databricks brings out-of-the-box features, including Azure Active Directory (AAD) integration, native data connectors, and integrated billing. It also brings three workloads (Jobs Compute, Job Light Compute, and all-purpose compute) on several VM instances tailored to your data analytics workflow. Learn about the Azure Databricks pricing.
Create Your Roadmap to an Intelligent Future with WinWire
Businesses often struggle to tap the highly useful insights because of ever increasing volume, velocity and variety of data. With Microsoft’s AI & Machine Learning platform, Azure based Big Data technologies and the entire SQL Server based Advanced Analytics platform you can gain critical insights to solve business problems and improve efficiency. However, the process of data mining for machine learning, to be executed smoothly and cost effectively requires strong experience in this field to achieve this transformation.
With our rich experience in Azure Data Platform Services, Data Mining for Machine Learning, we would create a roadmap for Azure cloud adoption and help build Big Data & ML solutions, Azure Databricks to simplify and empower your complex business decisions. Talk to us today.