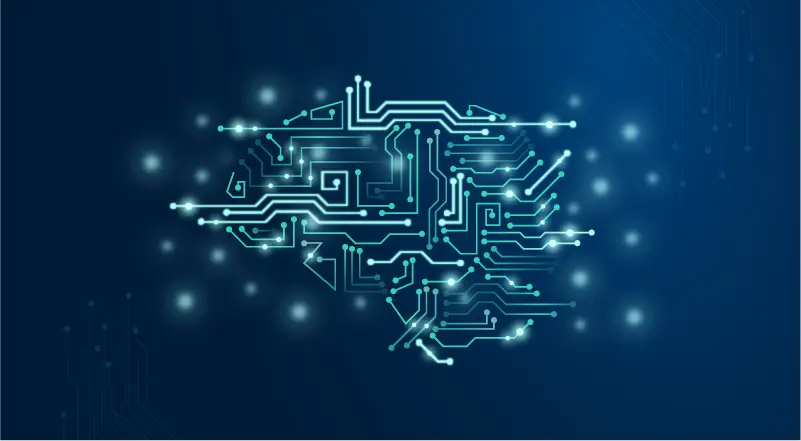
Enterprises across industry verticals are swamped in data, some of it structured but a lot of it is disparate and unstructured. A recent International Data Group study estimates that roughly 90 percent of the data generated today is unstructured. Using data to glean intelligence has been a successful trend, but in the recent past, AI and Machine Learning is helping to manage risk not by looking at organized data, but by evaluating unstructured data. This has been made possible by cognitive technologies such as natural language processing (NLP) which leverages advanced analytics to cull out intelligence from disparate data.
Samir Hans, advisory principal forensics & Investigations, Deloitte Transactions and Business Analytics says, “Many organizations have done a pretty good job of analyzing and interrogating structured data, but if you dump some contracts and medical literature and relevant raw material together, that’s unstructured, and the ability to analyze that data is the upside of cognitive”.
For instance, financial institutions regularly use data patterns to sniff out fraud. But then data will only show results of anomalies. It requires human’s intervention to analyze those anomalies. ‘What if’ just like the human mind, the system can learn from every anomaly instance and highlight those instances which resemble the anomaly? This is what AI & Machine Learning can do for you.
But then, immaterial of the size of an organization, tons of data are being disseminated from various business systems, databases, IoT and external sources. How does one make any intelligence out of it? Does this require complex data analysis and data scientists to unravel it?
In the absence of data scientists or processes in place how best to unravel data?
Often, companies take sample data, construct a model or use a tool, and at other times they build a solution and use it after testing to derive some learning. However, there is no systematic feeding of data, and therefore these solutions don’t scale to provide real, uncomplicated intelligence. Thus, such systems are good learning examples, but not workable solutions in the long run. Here are the steps on how you can derive intelligence from data:
1) Inculcate processes for data storage and retrieval
Organizations should follow a process to make AI work for them. The first step starts with identifying all the different data sources. Within the ocean of existing data, they need to identify which sources are unique and can be leveraged for decision making. This path to AI is rock solid. It establishes the foundation for actual AI.
2) Identify data sources that will result in meaningful AI
Taking sample data to form AI frameworks can result in untrue results. Larger the data, more varied its sources and information, stronger the chances of finding predictable patterns. But how does an organization determine which data sources need to be tapped? What if the data history stretches back in time? Or if you have just started the process of collecting data, where do you begin collecting it?
3) Standardize data format
Another challenge with data sources could be the format in which it exists, the more disparate and disorganized, the more challenging the path. It’s imperative to standardize the data format using a common data model (CDM). CDM provides well-defined, modular business entities such as product, lead, contact, etc., It also appears that CDM is evolving as part of the Open Data Initiative, a joint-development vision by Microsoft, Adobe, and SAP.When data has been cleaned and organized, then one should adopt tools such as Power BI to get insights and line of business tools.
4) Data democratization
Finally, when one applies AI on top of BI, we begin to understand the significance of the data. AI will cluster the data and compare it with other BI data to identify the emerging trends. When applied across a common process and scenario, AI gains strength and provides meaningful intelligence for adoption across the enterprise.
Self-service AI with Microsoft
All the steps till now can be managed by an enterprise without the help of a data scientist. Power BI (suite of business analytics tools to analyze data and share insights) effortlessly integrates with custom built Machine learning models using data visualizations that allow enterprises to create advanced analytics leveraging historic data.
Also, it’s advisable to run ‘What if’ Analysis, where you can run scenarios and scenario type on the data gathered. By implementing What if Analysis, it enables you to predict risk, identify problems, and develop strategies to mitigate risks. Implementing this in your models and Power BI reports enables you to create advanced analytics that can be classified as prediction or forecasting of what ‘could’ happen in the future.
AI majorly assists in data exploration, finding patterns to help the business drive better decisions. Power BI, Microsoft’s data visualization tool has recently incorporated several new AI features available in preview, allowing enterprises to make intelligent decision:
- Capabilities such as image recognition and text analytics
- Creates machine learning models directly in Power BI by leveraging automated machine learning
- Seamless integration of Azure Machine Learning within Power BI
- Methodical key driver analysis creation influencing key business metrics
These new AI capabilities pioneered in Azure doesn’t require a code. This allows the Power BI users to derive data-driven insights that helps to drive a better business outcome.
With Power BI, it’s possible for all to make better decisions and avoid risks based on data through visualization and a comprehensive dashboard. AI sifts through data to analyze patterns and help to drive business results and reduces risk. Traditionally, AI scientists helped with this, but now with Power BI being democratized. There is no need for coding, more importantly.
In the past, Power BI had included some AI capabilities such as NLP, however, in Azure Cognitive Services, this has been enhanced greatly to provide a strong path to extract actionable information from several sources which includes the documents, images, and the social media.
How WinWire Helped Leading Construction Company Streamline their Bidding Process by Leveraging Risk Modeling Solution with Predictive Analytics.
WinWire helped one of the leading construction company reduce human dependency and allowed them to streamline the bidding process by implementing a Risk Modeling Solution with Predictive Analytics. It focused on providing actionable information by leveraging historic data, interactive dashboards, What-IF analysis, and the Machine Learning model to identify potential problems and risks, developed strategies to mitigate risks which reduced human dependency and ensured a streamlined bidding process for the client.
Imperative to Have a Technology Partner
Even with all the tools and the ease with which one can use AI in the enterprise, there is the need for a partner who is sound in data management, has expertise in Power BI and a strong understanding of Microsoft Azure AI. For organizations, there are so many data entry points, that applying AI needs intervention.
As a proud Gold partner for Microsoft Data Platform and Analytics, WinWire’s Advanced Analytical solutions are generating tremendous value for organizations. With extensive proven expertise on Azure AI and machine learning technologies, we help organizations apply data science techniques, such as machine learning, AI, and NLP, to maximize revenue, reduce risk and optimize costs allowing them to stay ahead of the competition.
Ask WinWire about our Envisioning & Planning Sessions on AI and Machine Learning to learn how we are supporting our customers help identify use cases and build solutions to create a competitive advantage.